Our State of AI Survey Report discovered that one of many high challenges entrepreneurs face when utilizing generative AI is its skill to be biased.
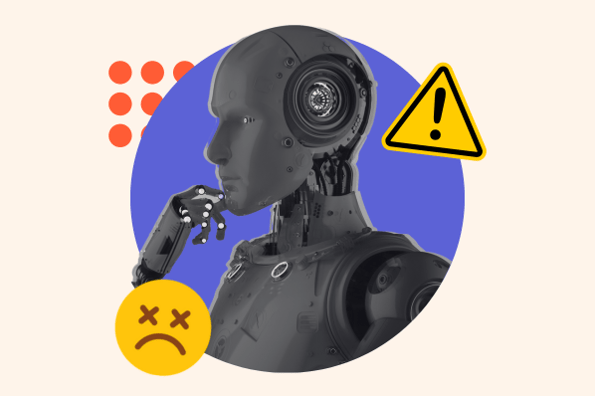
And entrepreneurs, gross sales professionals, and customer support folks report hesitating to make use of AI instruments as a result of they’ll generally produce biased data.
It’s clear that enterprise professionals are fearful about AI being biased, however what makes it biased within the first place? On this put up, we’ll talk about the potential for hurt in utilizing AI, examples of AI being biased in actual life, and the way society can mitigate potential hurt.
What’s AI bias?
AI bias is the concept that machine studying algorithms might be biased when finishing up their programmed duties, like analyzing information or producing content material). AI is often biased in ways in which uphold dangerous beliefs, like race and gender stereotypes.
In accordance with the Synthetic Intelligence Index Report 2023, AI is biased when it produces outputs that reinforce and perpetuate stereotypes that hurt particular teams. AI is truthful when it makes predictions or outputs that don’t discriminate or favor any particular group.
Along with being biased in prejudice and stereotypical beliefs, AI can be biased due to:
- Pattern choice, the place the information it makes use of isn’t consultant of whole populations, so its predictions and suggestions can’t be generalized or utilized to teams ignored
- Measurement, the place the information assortment course of is biased, main AI to make biased conclusions.
How does AI bias replicate society’s bias?
AI is biased as a result of society is biased.
Since society is biased, a lot of the information AI is educated on accommodates society’s biases and prejudices, so it learns these biases and produces outcomes that uphold them. For instance, a picture generator requested to create a picture of a CEO would possibly produce photos of white males due to the historic bias in unemployment within the information it realized from.
As AI turns into extra commonplace, a concern amongst many is that it has the potential to scale the biases already current in society which are dangerous to many alternative teams of individuals.
AI Bias Examples
The AI, Algorithmic, and Automation Incidents Controversies Repository (AIAAIC) says that the variety of newly reported AI incidents and controversies was 26 instances better in 2021 than in 2012.
Let’s go over some examples of AI bias.
Mortgage approval charges are a terrific instance of prejudice in AI. Algorithms have been discovered to be 40-80% extra more likely to deny debtors of shade as a result of historic lending information disproportionately reveals minorities being denied loans and different monetary alternatives. The historic information teaches AI to be biased with every future software it receives.
There’s additionally potential for pattern measurement bias in medical fields. Say a physician makes use of AI to research affected person information, uncover patterns, and description care suggestions. If that physician primarily sees White sufferers, the suggestions aren’t based mostly on a consultant inhabitants pattern and won’t meet everybody’s distinctive medical wants.
Some companies have algorithms that end in real-life biased decision-making or have made the potential for it extra seen.
1. Amazon’s Recruitment Algorithm
Amazon constructed a recruitment algorithm educated on ten years of employment historical past information. The info mirrored a male-dominated workforce, so the algorithm realized to be biased in opposition to functions and penalized resumes from ladies or any resumes utilizing the phrase “ladies(‘s).”
2. Twitter Picture Cropping
A viral tweet in 2020 confirmed that Twitter’s algorithm favored White faces over Black ones when cropping footage. A White consumer repeatedly shared footage that includes his face and that of a Black colleague and different Black faces in the identical picture, and it was persistently cropped to point out his face in picture previews.
Twitter acknowledged the algorithm’s bias and stated, “Whereas our analyses thus far haven’t proven racial or gender bias, we acknowledge that the way in which we mechanically crop pictures means there’s a potential for hurt. We should always’ve completed a greater job of anticipating this risk after we have been first designing and constructing this product.”
3. Robotic’s Racist Facial Recognition
Scientists just lately performed a examine asking robots to scan folks’s faces and categorize them into completely different bins based mostly on their traits, with three bins being medical doctors, criminals, and homemakers.
The robotic was biased in its course of and most frequently recognized ladies as homemakers, Black males as criminals, Latino males as janitors, and ladies of all ethnicities have been much less more likely to be picked as medical doctors.
4. Intel and Classroom Expertise’s Monitoring Software program
Intel and Classroom Expertise’s Class software program has a function that screens college students’ faces to detect feelings whereas studying. Many have stated completely different cultural norms of expressing emotion as a excessive likelihood of scholars’ feelings being mislabeled.
If academics use these labels to speak with college students about their degree of effort and understanding, college students might be penalized over feelings they’re not really displaying.
What might be completed to repair AI bias?
AI ethics is a sizzling matter. That is comprehensible as a result of AI’s bias has been demonstrated in actual life in many alternative methods.
Past being biased, AI can unfold damaging misinformation, like deepfakes, and generative AI instruments may even produce factually incorrect data.
What might be completed get a greater grasp on AI and scale back the potential bias?
- Human oversight: Folks can monitor outputs, analyze information, and make corrections when bias is displayed. For instance, entrepreneurs pays particular consideration to generative AI outputs earlier than utilizing them in advertising supplies to make sure they’re truthful.
- Assess the potential for bias: Some use instances for AI have the next potential for being prejudiced and dangerous to particular communities. On this case, folks can take the time to evaluate the chance of their AI producing biased outcomes, like banking establishments utilizing traditionally prejudiced information.
- Investing in AI ethics: One of the crucial vital methods to scale back AI bias is for there to be continued funding into AI analysis and AI ethics, so folks can devise concrete methods to scale back it.
- Diversifying AI: Having various views in AI helps create unbiased practices as folks carry their very own lived experiences. A various and consultant subject brings extra alternatives for folks to acknowledge the potential for bias and take care of it earlier than hurt is brought about.
- Acknowledge human bias: All people have the potential for bias, whether or not from a distinction in lived expertise or affirmation bias throughout analysis. Folks utilizing AI can acknowledge their biases to make sure their AI isn’t biased, like researchers ensuring their pattern sizes are consultant.
- Being clear: Transparency is all the time vital, particularly with new applied sciences. Folks can construct belief and understanding with AI by merely making it recognized after they use AI, like including a be aware beneath an AI-generated information article.
It is very potential to make use of AI responsibly.
AI and curiosity in AI are solely rising, so one of the best ways to remain on high of the potential for hurt is to remain knowledgeable on the way it can perpetuate dangerous biases and take motion to make sure your use of AI would not add extra gasoline to the hearth.
Need to be taught extra about synthetic intelligence? Try this studying path.